CHTC Partners Using CHTC Technologies and Services
IceCube and Francis Halzen
Francis Halzen, principal investigator of IceCube and the Hilldale and Gregory Breit Distinguished Professor of Physics.
IceCube has transformed a cubic kilometer of natural Antarctic ice into a neutrino detector. We have discovered a flux of high-energy neutrinos of cosmic origin, with an energy flux that is comparable to that of high-energy photons. We have also identified its first source: on September 22, 2017, following an alert initiated by a 290-TeV neutrino, observations by other astronomical telescopes pinpointed a flaring active galaxy, powered by a supermassive black hole. We study the neutrinos themselves, some with energies exceeding by one million those produced by accelerators. The IceCube Neutrino Observatory is managed and operated by the Wisconsin IceCube Astroparticle Physics Center (WIPAC) in the Office of the Vice Chancellor of Graduate Education and Research and funded by a cooperative agreement with the National Science Foundation. We have used CHTC and the Open Science Pool for over a decade to perform all large-scale data analysis tasks and generate Monte Carlo simulations of the instrument's performance. Without CHTC and OSP resources we would simply be unable to make any of IceCube's groundbreaking discoveries. Francis Halzen is the Principal Investigator of IceCube. See the IceCube web site for project details.
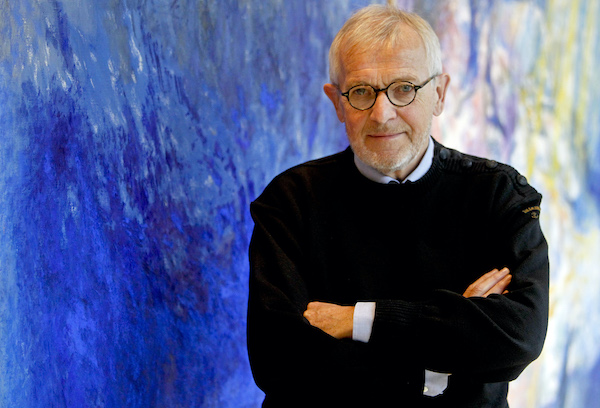
David O’Connor
David H. O’Connor, Ph.D., UW Medical Foundation (UWMF) Professor, Department of Pathology and Laboratory Medicine at the AIDS Vaccine Research Laboratory.
Computational workflows that analyze and process genomics sequencing data have become the standard in Virology and genomics research. The resources provided by CHTC allow us to scale up the amount of sequence analysis performed while decreasing sequence processing time. An example of how we use CHTC is generating consensus sequences for COVID-19 samples. Part of this is a step that separates and sorts a multisample sequencing run into individual samples, maps the reads of these individual samples to a reference sequence, and then forms a consensus sequence for each sample. Simultaneously, different metadata and other accessory files are generated on a per-sample basis, and data is copied to and from local machines. This workflow is cumbersome when there are, for example, 4 sequencing runs with each run containing 96 samples. CHTC allows us to cut the processing time from 16 hours to 40 minutes due to the distribution of jobs to different CPUs. Overall, being able to use CHTC resources gives us a major advantage in producing results faster for large-scale and/or time-sensitive projects.
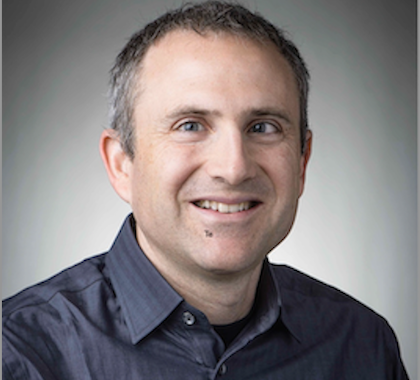
Small Molecule Screening Facility and Spencer Ericksen
Spencer Ericksen, Scientist II at the Small Molecule Screening Facility, part of the Drug Development Core – a shared resource in the UW Carbone Cancer Center.
I have been working on computational methods for predicting biomolecular recognition processes. The motivation is to develop reliable models for predicting binding interactions between drug-like small molecules and therapeutic target proteins. Our team at SMSF works with campus investigators on early-stage academic drug discovery projects. Computational models for virtual screening could prioritize candidate molecules for faster, cheaper focused screens on just tens of compounds. To perform a virtual screen, the models evaluate millions to billions of molecules, a computationally daunting task. But CHTC facilitators have been with us through every obstacle, helping us to effectively scale through parallelization over HTC nodes, matching appropriate resources to specific modeling tasks, compiling software, and using Docker containers. Moreover, CHTC provides access to vast and diverse compute resources.
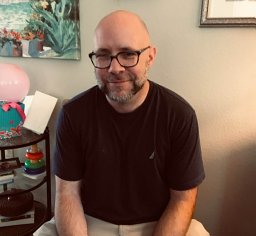
xDD project and Shanan Peters
Shanan Peters, project lead for xDD, Dean L. Morgridge Professor of Geology, Department of Geoscience.
Shanan’s primary research thrust involves quantifying the spatial and temporal distribution of rocks in the Earth’s crust in order to constrain the long-term evolution of life and Earth’s surface environment. Compiling data from scientific publications is a key component of this work and Peters and his collaborators are developing machine reading systems deployed over the xDD digital library ad cyberinfrastructure hosted in the CHTC for this purpose.
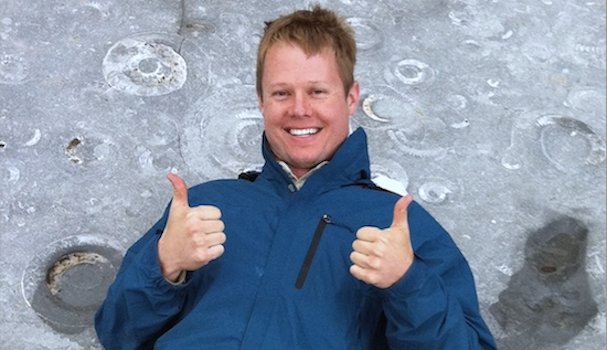
Natalia de Leon
Natalia de Leon, Professor of Agronomy, Department of Agronomy.
The goal of her research is to identify efficient mechanisms to better understand the genetic constitution of economically relevant traits and to improve plant breeding efficiency. Her research integrates genomic, phenomic, and environmental information to accelerate translational research for enhanced sustainable crop productivity.
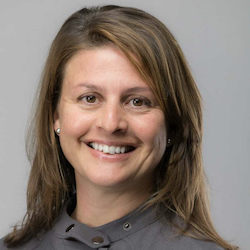
Susan Hagness
In our research we're working on a novel computational tool for THz-frequency characterization of materials with high carrier densities, such as highly-doped semiconductors and metals. The numerical technique tracks carrier-field dynamics by combining the ensemble Monte Carlo simulator of carrier dynamics with the finite-difference time-domain technique for Maxwell's equations and the molecular dynamics technique for close-range Coulomb interactions. This technique is computationally intensive and each test runs long enough (12-20 hours) that our group's cluster isn't enough. This is why we think CHTC can help, to let us run more jobs than we're able to run now.
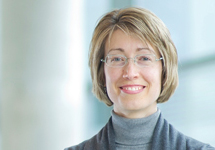
Joao Dorea
Joao Dorea, Assistant Professor in the Department of Animal and Dairy Sciences/Department of Biological Systems Engineering.
The Digital Livestock Lab develops research focused on high-throughput phenotyping strategies to optimize farm management decisions. Our research group is interested in the large-scale development and implementation of computer vision systems, wearable sensors, and infrared spectroscopy (NIR and MIR) to monitor animals in livestock systems. We have a large computer vision system implemented in two UW research farms that generate large datasets. With the help of CHTC, we can train deep learning algorithms with millions of parameters using large image datasets and evaluate their performance in farm settings in a timely manner. We use these algorithms to monitor animal behavior, growth development, social interaction, and to build predictive models for early detection of health issues and productive performance. Without access to the GPU cluster and the facilitation made by CHTC, we would not be able to quickly implement AI technologies in livestock systems.
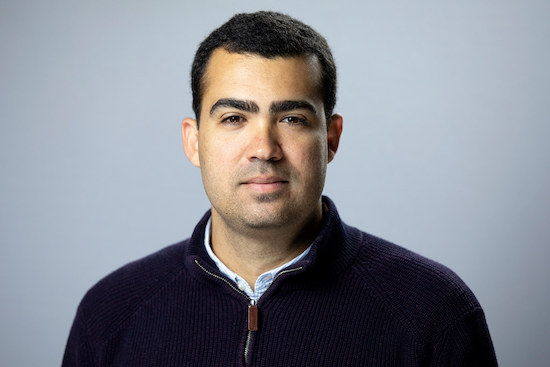
Paul Wilson
Paul Wilson, head of The Computational Nuclear Engineering Research Group (CNERG), the Grainger Professor for Nuclear Engineering, and the current chair of the Department of Engineering Physics.
CNERG’s mission is to foster the development of new generations of nuclear engineers and scientists through the development and deployment of open and reliable software tools for the analysis of complex nuclear energy systems. Our inspiration and motivation come from performing those analyses on large, complex systems. Such simulations require ever-increasing computational resources and CHTC has been our primary home for both HPC and HTC computation for nearly a decade. In addition to producing our results faster and without the burden of administering our computer hardware, we rely on CHTC resources to demonstrate performance improvements that arise from our methods development. The role-defining team of research computing facilitators has ensured a smooth onboarding of each of them and helped them find the resources they need to be most effective.
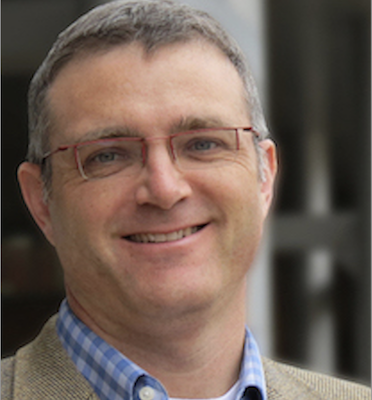
Biomagnetic Resonance Data Bank
The Biomagnetic Resonance Data Bank (BMRB) is headquarted within UW-Madison's National Magnetic Resonance Facility at Madison (NMRFAM) and uses the CHTC for research in connection with the Biological Magnetic Resonance Data Bank (BMRB).
CMS LHC Compact Muon Solenoid
The UW team participating in the Compact Muon Solenoid (CMS) experiment analyzes petabytes of data from proton-proton collisions in the Large Hadron Collider (LHC). We use the unprecedented energies of the LHC to study Higgs Boson signatures, Electroweak Physics, and the possibility of exotic particles beyond the Standard Model of Particle Physics. Important calculations are also performed to better tune the experiment's trigger system, which is responsible for making nanosecond-scale decisions about which collisions in the LHC should be recorded for further analysis.
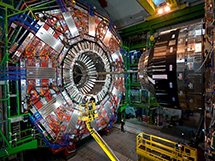
Atlas Experiment
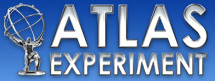
Phil Townsend
Professor Phil Townsend of Forestry and Wildlife Ecology says Our research (NASA & USDA Forest Service funded) strives to understand the outbreak dynamic of major forest insect pests in North America through simulation modeling. As part of this effort, we map forest species and their abundance using multi-temporal Landsat satellite data. My colleagues have written an automatic variable selection routine in MATLAB to preselect the most important image variables to model and map forest species abundance. However, depending on the number of records and the initial variables, this process can take weeks to run. Hence, we seek resources to speed up this process.
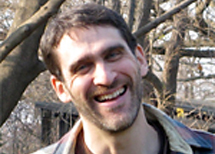
Barry Van Veen
The bio-signal processing laboratory develops statistical signal processing methods for biomedical problems. We use CHTC for casual network modeling of brain electrical activity. We develop methods for identifying network models from noninvasive measures of electric/ magnetic fields at the scalp, or invasive measures of the electric fields at or in the cortex, such as electrocorticography. Model identification involves high throughput computing applied to large datasets consisting of hundreds of spatial channels each containing thousands of time samples.
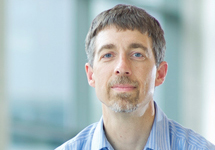